The meaning of life.
Ongoing analyses.
Initial analysis utilizing Amazon Mechanical Turk workers.
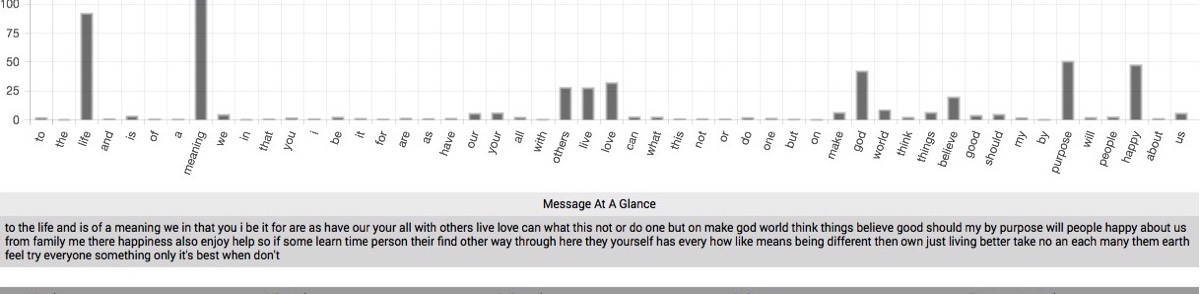
1. Acknowledge and refine your attitude.
2. Over the course of a sentence or two, please tell us in your own words how it is that you came to your perspective on the meaning of life.
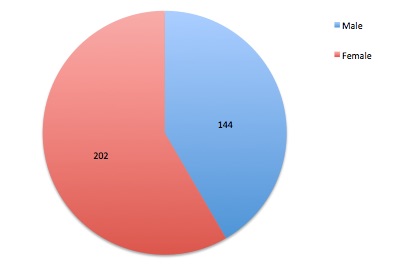
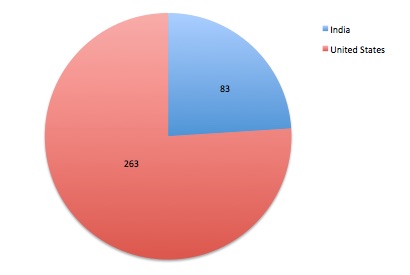
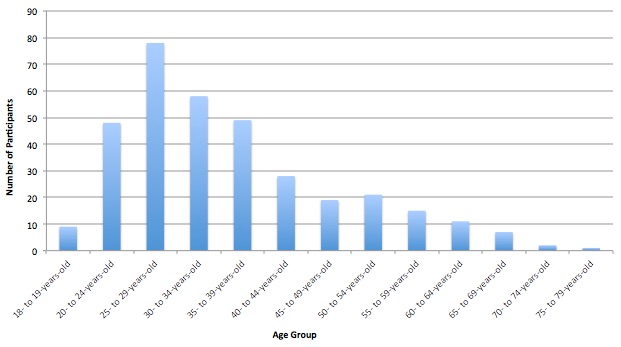
Such scores indicate that overall, the participants expressed an average level of verbosity and cognitive functioning in their responses. Word counts, sentence counts, average sentence length, average response length, and Flesch scores for each separate question are analyzed in more detail in step 2.2.1.
2. Get a sense of the whole.
In Table 1, the words, we, our, and us can be combined to form one gist, which would be labeled we. Likewise, the words happy and happiness can be combined to form a gist labeled happy. In Table 2, the words, I, my, and me can be combined to form a gist labeled I, while the words, live and living can be combined to form the gist live, and the words experience and experiences can be combined to form the gist experience.
Word | # Sample | % Sample | % Corpus | Over- representation |
life | 733 | 4.146 | 0.045 | 92.133 |
meaning | 363 | 2.053 | 0.008 | 256.625 |
we | 324 | 1.833 | 0.38 | 4.824 |
our | 126 | 0.713 | 0.122 | 5.844 |
your | 116 | 0.656 | 0.104 | 6.308 |
others | 105 | 0.594 | 0.021 | 28.286 |
live | 104 | 0.588 | 0.021 | 28 |
love | 103 | 0.583 | 0.018 | 32.389 |
make | 78 | 0.441 | 0.067 | 6.582 |
god | 75 | 0.424 | 0.01 | 42.4 |
world | 75 | 0.424 | 0.047 | 9.021 |
things | 71 | 0.402 | 0.061 | 6.59 |
believe | 71 | 0.402 | 0.02 | 20.1 |
good | 69 | 0.39 | 0.094 | 4.149 |
should | 68 | 0.385 | 0.077 | 5 |
purpose | 63 | 0.356 | 0.007 | 50.857 |
happy | 59 | 0.334 | 0.007 | 47.714 |
us | 57 | 0.322 | 0.054 | 5.963 |
family | 54 | 0.305 | 0.042 | 7.262 |
happiness | 52 | 0.294 | 0.001 | 294 |
enjoy | 49 | 0.277 | 0.012 | 23.083 |
help | 49 | 0.277 | 0.029 | 9.552 |
learn | 45 | 0.255 | 0.009 | 28.333 |
person | 44 | 0.249 | 0.019 | 13.105 |
find | 42 | 0.238 | 0.039 | 6.103 |
Word | # Sample | % Sample | % Corpus | Over- representation |
I | 601 | 5.15 | 1.056 | 4.877 |
life | 296 | 2.536 | 0.045 | 56.356 |
my | 256 | 2.193 | 0.168 | 13.054 |
me | 109 | 0.934 | 0.085 | 10.988 |
meaning | 84 | 0.72 | 0.008 | 90 |
things | 68 | 0.583 | 0.061 | 9.557 |
came | 67 | 0.574 | 0.022 | 26.091 |
perspective | 56 | 0.48 | 0.004 | 120 |
through | 51 | 0.437 | 0.065 | 6.723 |
others | 47 | 0.403 | 0.021 | 19.19 |
god | 43 | 0.368 | 0.01 | 36.8 |
am | 41 | 0.351 | 0.02 | 17.55 |
believe | 36 | 0.308 | 0.02 | 15.4 |
family | 35 | 0.3 | 0.042 | 7.143 |
live | 34 | 0.291 | 0.021 | 13.857 |
world | 34 | 0.291 | 0.047 | 6.191 |
living | 34 | 0.291 | 0.014 | 20.786 |
I’ve | 30 | 0.257 | 0.052 | 4.942 |
experience | 28 | 0.24 | 0.013 | 18.462 |
experiences | 28 | 0.24 | 0.003 | 80 |
feel | 28 | 0.24 | 0.024 | 10 |
love | 27 | 0.231 | 0.018 | 12.833 |
happy | 26 | 0.223 | 0.007 | 31.857 |
always | 24 | 0.206 | 0.038 | 5.421 |
thought | 23 | 0.197 | 0.03 | 6.567 |
Word | # Sample | % Sample | % Corpus | Overrepresentation |
---|---|---|---|---|
life | 733 | 4.146 | .045 | 92.133 |
meaning | 363 | 2.053 | .008 | 256.625 |
we | 324 | 1.833 | .38 | 4.824 |
our | 126 | .713 | .122 | 5.844 |
your | 116 | .656 | .104 | 6.308 |
others | 105 | .594 | .021 | 28.286 |
live | 104 | .588 | .021 | 28 |
love | 103 | .583 | .018 | 32.389 |
make | 78 | .441 | .067 | 6.582 |
god | 75 | .424 | .01 | 42.4 |
world | 75 | .424 | .047 | 9.021 |
things | 71 | .402 | .061 | 6.59 |
believe | 71 | .402 | .02 | 20.1 |
good | 69 | .39 | .094 | 4.149 |
should | 68 | .385 | .077 | 5 |
purpose | 63 | .356 | .007 | 50.857 |
happy | 59 | .334 | .007 | 47.714 |
us | 57 | .322 | .054 | 5.963 |
family | 54 | .305 | .042 | 7.262 |
happiness | 52 | .294 | .001 | 294 |
enjoy | 49 | .277 | .012 | 23.083 |
help | 49 | .277 | .029 | 9.552 |
learn | 45 | .255 | .009 | 28.333 |
person | 44 | .249 | .019 | 13.105 |
find | 42 | .238 | .039 | 6.103 |
Word | # Sample | % Sample | % Corpus | Overrepresentation |
---|---|---|---|---|
I | 601 | 5.15 | 1.056 | 4.877 |
life | 296 | 2.536 | .045 | 56.356 |
my | 256 | 2.193 | .168 | 13.054 |
me | 109 | .934 | .085 | 10.988 |
meaning | 84 | .72 | .008 | 90 |
things | 68 | .583 | .061 | 9.557 |
came | 67 | .574 | .022 | 26.091 |
perspective | 56 | .48 | .004 | 120 |
through | 51 | .437 | .065 | 6.723 |
others | 47 | .403 | .021 | 19.19 |
god | 43 | .368 | .01 | 36.8 |
am | 41 | .351 | .02 | 17.55 |
believe | 36 | .308 | .02 | 15.4 |
family | 35 | .3 | .042 | 7.143 |
live | 34 | .291 | .021 | 13.857 |
world | 34 | .291 | .047 | 6.191 |
living | 34 | .291 | .014 | 20.786 |
I’ve | 30 | .257 | .052 | 4.942 |
experience | 28 | .24 | .013 | 18.462 |
experiences | 28 | .24 | .003 | 80 |
feel | 28 | .24 | .024 | 10 |
love | 27 | .231 | .018 | 12.833 |
happy | 26 | .223 | .007 | 31.857 |
always | 24 | .206 | .038 | 5.421 |
thought | 23 | .197 | .03 | 6.567 |
When the metadata for Question 1 are compared across the classes of variables acquired, we find no substantial variation (see Table 3). However, we do note that there is a tendency for participants from India to express statements that are somewhat less complex than those from the United States, and that women tend to be somewhat more verbose than men.
Female | Male | India | U.S. | |
Word Count | 10710 | 6968 | 3193 | 14485 |
Sentence Count | 729 | 486 | 264 | 951 |
Sentence Length | 14.69 | 14.33 | 12.09 | 15.23 |
Average Response Length | 53.02 | 48.39 | 38.47 | 55.08 |
Average Sentences per Response | 3.61 | 3.38 | 3.18 | 3.62 |
Flesch Reading Ease | 77.04 | 73.8 | 79.14 | 74.87 |
Flesch-Kincaid Grade Level | 6.16 | 6.53 | 5.22 | 6.6 |
Variable | Word Count | Sentence Count | Sentence Length | Average Response Length | Average Sentences per Response | Flesch Reading Ease Score | Flesch-Kincaid Grade Level |
---|---|---|---|---|---|---|---|
Female | 10710 | 729 | 14.69 | 53.02 | 3.61 | 77.04 | 6.16 |
Male | 6968 | 486 | 14.33 | 48.39 | 3.38 | 73.8 | 6.53 |
India | 3193 | 264 | 12.09 | 38.47 | 3.18 | 79.14 | 5.22 |
U.S. | 14485 | 951 | 15.23 | 55.08 | 3.62 | 74.87 | 6.6 |
When the metadata for Question 2 are compared across the classes of variables acquired, we find no substantial variation (see Table 4). However, and as with Question 1, we do note that there is a tendency for participants from India to express statements that are somewhat less complex than those from the United States, and that women tend to be somewhat more verbose than men.
Female | Male | India | U.S. | |
Word Count | 7371 | 4300 | 1856 | 9815 |
Sentence Count | 465 | 285 | 147 | 603 |
Sentence Length | 15.85 | 15.08 | 12.63 | 16.28 |
Average Response Length | 36.49 | 29.86 | 38.47 | 37.32 |
Average Sentences per Response | 2.3 | 1.98 | 3.05 | 2.29 |
Flesch Reading Ease | 73.33 | 70.6 | 78.1 | 71.08 |
Flesch-Kincaid Grade Level | 6.97 | 7.16 | 5.5 | 7.39 |
Variable | Word Count | Sentence Count | Sentence Length | Average Response Length | Average Sentences per Response | Flesch Reading Ease Score | Flesch-Kincaid Grade Level |
---|---|---|---|---|---|---|---|
Female | 7371 | 465 | 15.85 | 36.49 | 2.3 | 73.33 | 6.97 |
Male | 4300 | 285 | 15.08 | 29.86 | 1.98 | 70.6 | 7.16 |
India | 1856 | 147 | 12.63 | 38.47 | 3.05 | 78.1 | 5.5 |
U.S. | 9815 | 603 | 16.28 | 37.32 | 2.29 | 71.08 | 7.39 |
3. Constitute parts by identifying meaning units.
Given that this is an initial analysis, we continue to divide meaning units by their most overt form: each response to a question is considered one complete unit of meaning. The sample continues, therefore, to be divided into two main parts, in which each correspond to the two questions asked of the participants. If desired or needed for increased specificity, in subsequent analyses we might consider separating the first sentence in each response from the other sentences in the response, and analyzing these as separate meaning units.
4. Transform data into revelatory statements.
With respect to our current project, when overrepresented gists are elaborated, several key concepts emerge for each question. Given that this is an initial demonstration of how to conduct a Quantitative Phenomenology with Raven’s Eye on this topic, we provide examples for the top ten concepts for each question.
Statements derived from associated concepts preceding the gist.
Statements derived from associated concepts following the gist.
… is to…
Statements derived from associated concepts preceding the gist.
Statements derived from associated concepts following the gist.
… came to my perspective…
5. Identify essential structure.
Notes.
2 As noted in Step 2.1 of performing a Quantitative Phenomenology with Raven’s Eye, a gist represents all of a given word’s applicable inflections found in the sample, but it assumes the label of the word’s most proportional inflection.
3 We limit the discussion in this section to illustrating how to associate the concept life. However, when we inspect the overrepresented concept meaning, (i.e., selecting the gist meaning in the main table, and then use the Word Association feature to inspect the two words that follow it in a sentence) reveals that approximately 80% of instances of the gist meaning are followed within two words by the concept life.